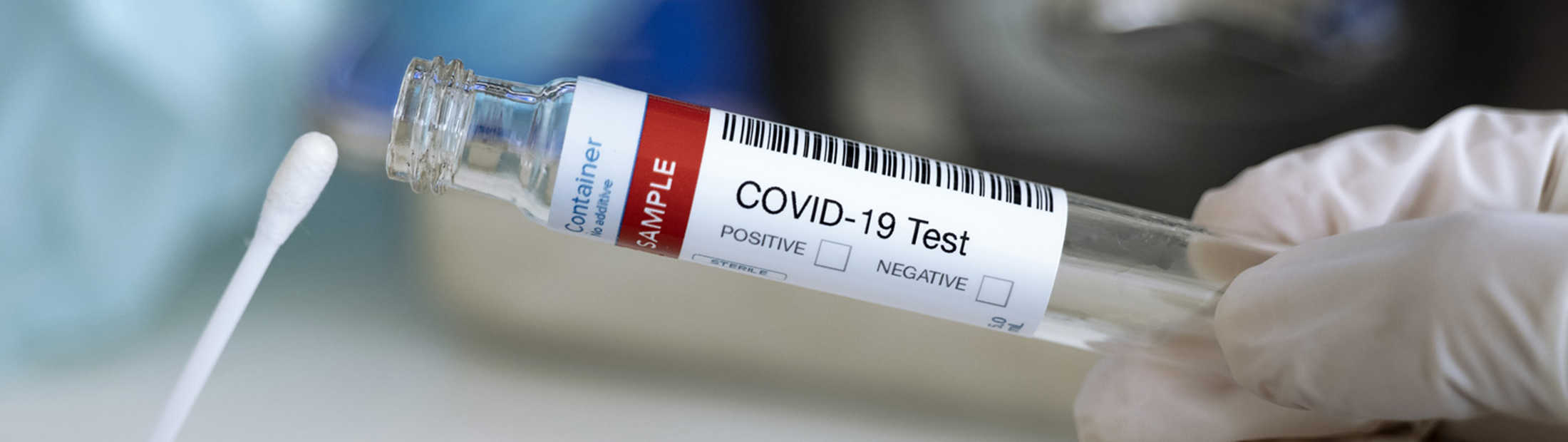
How big data could optimize COVID-19 testing
Microsoft-supported project to coordinate site locations, supply distribution.
Microsoft-supported project to coordinate site locations, supply distribution.
The shift in COVID-19 caseloads around the U.S. in recent weeks has scrambled supply and demand in the nation’s testing infrastructure. Even though testing capacity has increased dramatically since spring, the hardest-hit geographical areas are seeing demand outstripping supply, while a few areas are actually reporting excess capacity.
Now, University of Michigan industrial operations and engineering associate professor Siqian Shen, with support from Microsoft’s AI for Health program, is stepping in to help, designing a cloud-based system that will analyze infection and testing supply chain data to better organize the logistics of testing. It will provide policymakers with data that can help them determine how to distribute tests and site mobile testing centers to make testing as efficient and accessible as possible.
“Without a centralized source of data, it’s very difficult to optimize testing and minimize the spread of COVID.” Shen said. “By pulling in daily updates on infection rates and anonymous demographic data, along with data from the testing supply chain, we’re building a mathematical computer model that will crunch that data and provide visualizations that could help policymakers optimize testing and reduce the spread of the disease.”
Shen said the model will be freely available to U.S. decisionmakers like federal, state and local governments as well as school districts that are weighing their options for reopening. The research team will build the model during the rest of the summer and aims to have it ready for use in the fall. The nuts and bolts of the model will be freely available, enabling other countries to adapt it for their needs.
“I think this system is likely to be most useful for the U.S., since we’re facing a lack of coordination of testing and disease management. But any country will be able to use the model to manage their testing systems. It may be useful for managing testing and control of other infectious diseases as well,” she said.
Later in 2020, the research team plans to expand the model’s capabilities further with an online appointment scheduling system that enables those seeking tests to easily find their nearest testing center, see the caseload at that particular center on any given day and evaluate accessibility options at different centers. Shen envisions that part of the system coming online in late 2020 or early 2021.
“Different people have different testing needs,” Shen said. “Some may need a facility that’s accessible to the disables, others may be in a high-risk group and want to visit during a time that’s less busy, and still others may be low-income and have limited transportation options. This system can help people meet those needs and also reduce crowding and increase efficiency at testing centers.”
Microsoft’s AI for Health program is supporting the project by providing free access to its Azure computing platform. The team will use freely available public data from the Michigan Institute for Clinical & Health Research (MICHR), as well as US census data and Google Maps data on the locations of testing facilities and testing equipment manufacturing facilities.