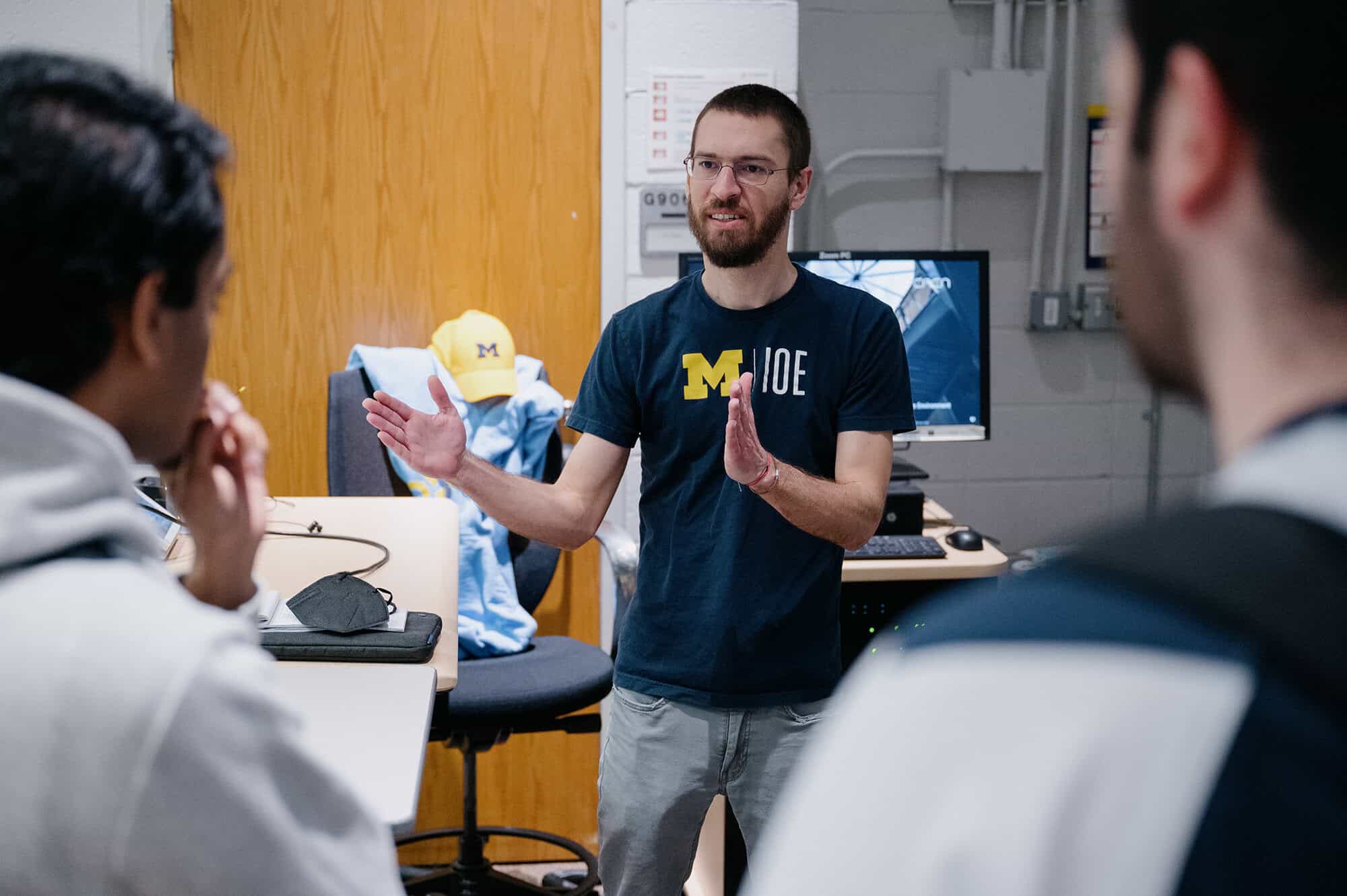
Albert S. Berahas wins Charles Broyden Prize
U-M IOE assistant professor recognized for advancements in machine learning optimization
U-M IOE assistant professor recognized for advancements in machine learning optimization
University of Michigan (U-M) Industrial and Operations Engineering (IOE) Assistant Professor Albert S. Berahas was awarded the Charles Broyden Prize for his research paper titled “Quasi-Newton methods for machine learning: forget the past, just sample.“
The award, announced on January 8, 2025, was presented by the Optimization Methods and Software Editorial Board and Taylor & Francis, in recognition of the best paper published in the journal during the previous year.
The Charles Broyden Prize, named after the mathematician and optimization expert, has been awarded annually since 2009. It honors innovative contributions to the field of optimization, with a focus on research that demonstrates outstanding originality and impact.
In the awarded paper, Berahas and his co-authors introduced new advancements in quasi-Newton methods for machine learning. Their research challenges traditional approaches and proposes novel strategies for solving optimization problems that arise in machine learning, by forgetting past and possibly stale information, and sampling new information as needed.
“It is an incredible honor to win this award, and it was completely unexpected,” said Berahas. “Charles George Broyden is a legend in optimization! I would like to thank my wonderful collaborators and the Optimization Methods and Software Editorial Board and Taylor & Francis.”
Prior to his time at U-M IOE, Berahas was a postdoctoral research fellow at Lehigh University and Northwestern University. He received his Ph.D. in Engineering Sciences and Applied Mathematics from Northwestern University. Berahas received his B.S.E. in Operations Research and Industrial Engineering from Cornell University and his MSc and Ph.D. in Engineering Sciences and Applied Mathematics from Northwestern University.
His research broadly focuses on designing, developing and analyzing algorithms for solving large-scale nonlinear optimization problems.